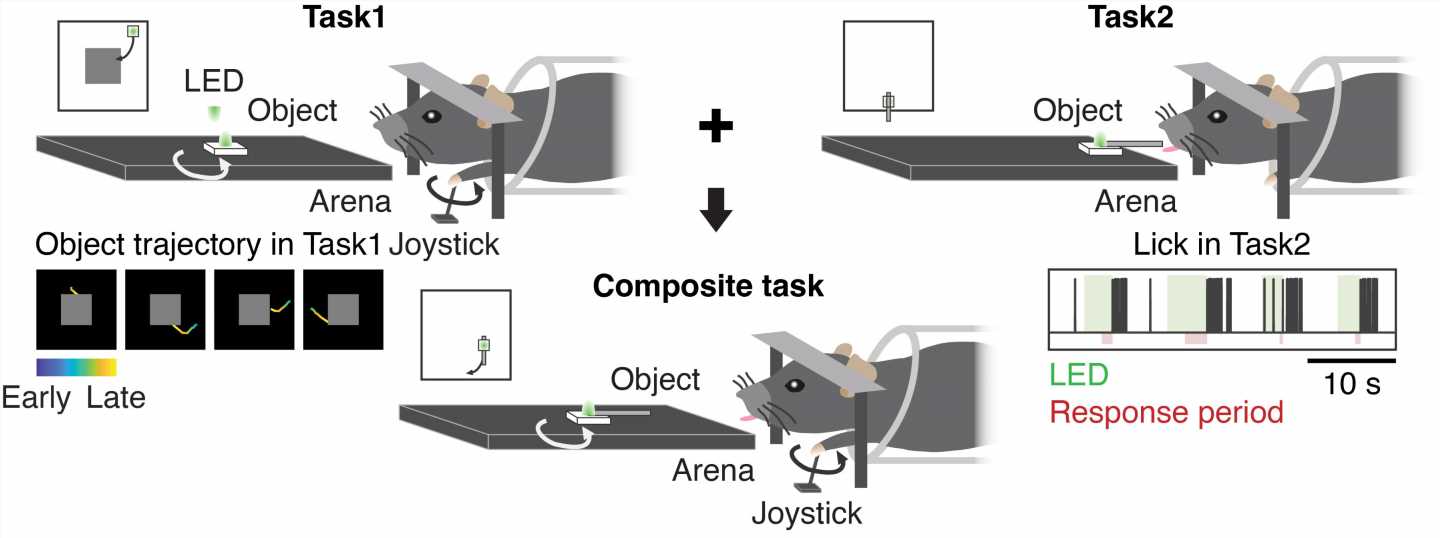
Artificial intelligence (AI) draws inspiration from current knowledge of the human brain, artificially replicating some of its learning processes and functions. The extent to which artificial agents successfully emulate the brain’s activity, however, is not yet fully understood.
Hiroshi Makino, a researcher at Lee Kong Chian School of Medicine, Nanyang Technological University in Singapore, recently carried out a study exploring the possible similarities in how the brain and artificial agents learn to solve new composite tasks. His findings, published in Nature Neuroscience, could have interesting implications for both AI and neuroscience research.
“Previous studies in psychology showed that humans and non-human animals combine pre-learned skills to expand their behavior repertoires,” Hiroshi Makino, the researcher who carried out the study, told Medical Xpress. “However, how the brain achieves this remains poorly understood. I was inspired by research in deep reinforcement learning (a subfield of AI research) studying the same problem, and empirically tested theoretical predictions derived from it by recording neural activity in the mouse brain.”
When solving a new task, AI agents extract skills acquired during pre-training and recombine these skills in a hierarchical fashion. Makino wanted to explore the possibility that the brain behaves in a similar way.
To do this, he first looked at how deep reinforcement learning agents learned to solve a new task. Subsequently, he compared this with the way in which mice tackled the same new task.
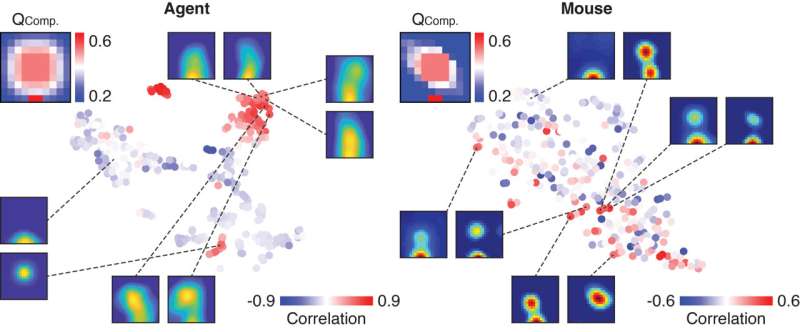
“As the mice solved a new problem by combining pre-acquired skills/knowledge, activity was recorded from single neurons in their brains,” Makino explained. “The resulting neural activity was compared with theoretical models derived from deep reinforcement learning where a simple arithmetic operation was used to combine values of pre-learned behaviors.”
Using a technique known as two-photon calcium imaging, Makino explored what happened in the mouse cortex, the brain region associated with executive functions and learning, as they tackled a new task using previously acquired skills. Interestingly, he observed neural representations of action values that resembled those created by a deep reinforcement learning algorithm when approaching a new composite task.
“I found similar activity patterns between the artificial agent trained with a deep reinforcement learning algorithm and the brain,” Makino said. “I think one of the major contributions of the study is the integration of neuroscience and deep reinforcement learning to identify a potential mechanism for how the brain composes a new behavior.”
Overall, the findings of Makino’s study suggest that when tackling a new task, the mammalian brain composes a new behavior through a simple arithmetic operation on previously acquired action-value representations with stochastic policies. These ideas were originated from the theoretical framework of deep reinforcement learning.
In the future, this recent study could pave the way for new studies linking neuroscientific observations to deep reinforcement learning research. These works could collectively enable the continuous improvement of artificially intelligent systems, while also potentially enhancing the present understanding of the mammalian brain.
“I now plan to study neural mechanisms of various domains of natural intelligence by applying theoretical concepts of deep reinforcement learning to neuroscience,” Makino added.
More information:
Hiroshi Makino, Arithmetic value representation for hierarchical behavior composition, Nature Neuroscience (2022). DOI: 10.1038/s41593-022-01211-5
Journal information:
Nature Neuroscience
Source: Read Full Article