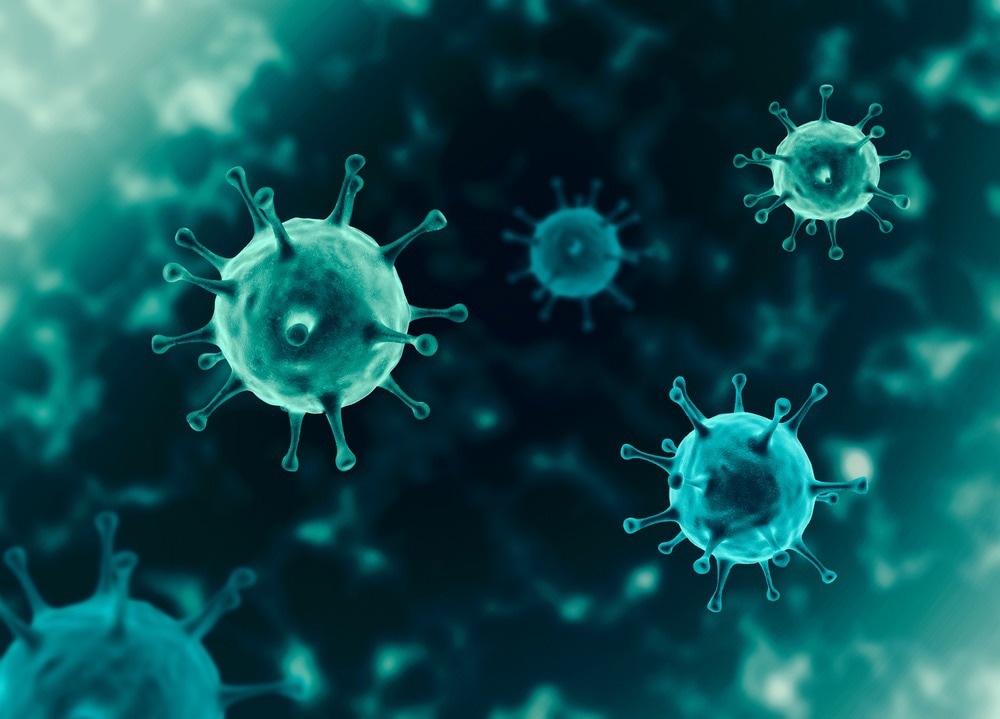
In a recent study published in PLOS One, researchers developed a causal model to analyze severe acute respiratory syndrome coronavirus 2 (SARS-CoV-2) viral load distribution as a function of patients’ age.
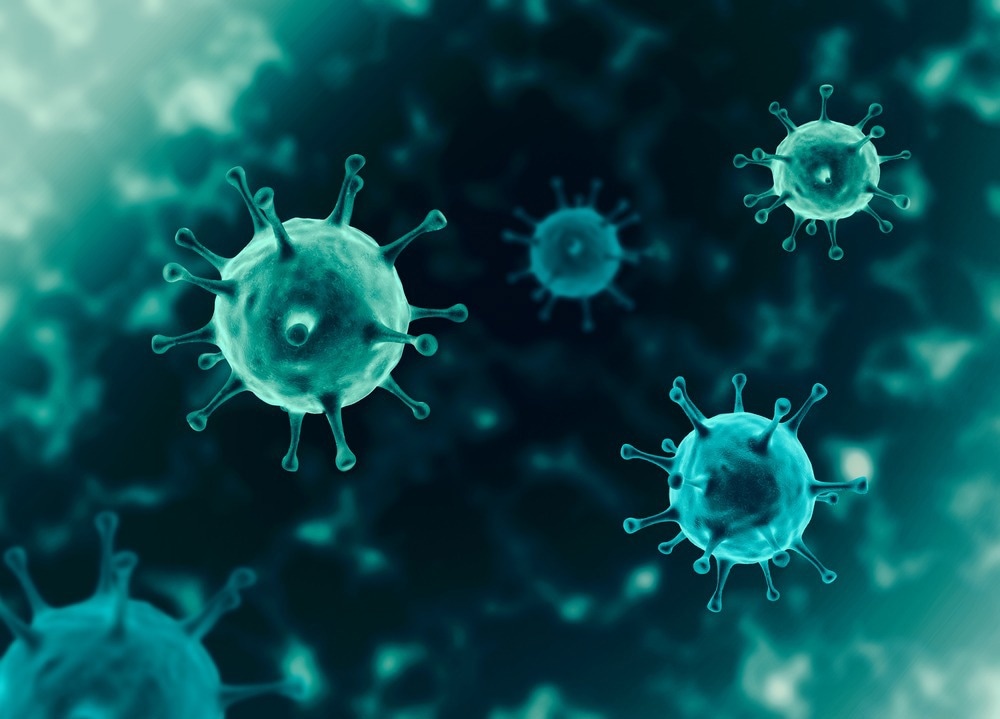
Background
The true extent to which adolescents and children get infected by SARS-CoV-2 is not well known. Their role in community transmission of SARS-CoV-2 is contingent on symptoms, viral load, behavior, susceptibility, and existing mitigation strategies. Viral load is the concentration of the virus in the upper airways and is typically expressed as viral RNA copies per milliliter of sample.
Viral load is inferred from a specimen’s cycle threshold (Ct) value in a reverse-transcription polymerase chain reaction (RT-PCR) test. Several studies have investigated whether children and adults exhibit differential viral loads during coronavirus disease 2019 (COVID-19). Viral load is a critical variable that could help predict the severity and mortality of COVID-19.
About the study
The present study examined viral load as a proxy for SARS-CoV-2 infectivity and re-analyzed age-stratified data previously reported by another research group through a non-parametric, Bayesian, and causal model. Since the COVID-19 outbreak, efforts have been made to identify whether individuals of specific age groups are more susceptible to infection than others.
To explain the SARS-CoV-2 viral load and age data, a model should integrate fundamental knowledge about the causal relationship between viral load and age. A non-parametric, causal model was developed and applied to the data. RT-PCR viral load data from the Charite Institute of Virology and Labor, Germany. These data were obtained with two PCR instruments – Roche Cobas 6800/8800 and Roche LightCycler 480 II.
The cobas dataset, denoted by dC, comprised approximately 2,200 data points, whereas the LC480 (dL) dataset comprised around 1,350 data points. The analyzed dataset consisted of indexed pairs of age (x) and log-viral load (y) for each of the ‘N’ infected patients. Two lower data-filtering thresholds for viral loads were defined – ymin (3.8) and y’min (5.4) and any data point with a viral load lower than ymin and y’min was discarded. The two datasets were first analyzed by excluding data below ymin and then below y’min.
Findings
The authors observed, in general, a descending trend in viral load probability distribution for all datasets and age groups. The dC dataset showed significant differences in the viral load for different age groups. The distribution had a distinct maximum for viral loads equal to or above 8 (in log units) for patients older than 60. The researchers noted that this was not a consequence of an over-fit of sample noise but was triggered by data. This meant that the differences in the real data were not just a shot noise effect.
For the causal structure x → y (age influences viral load), there was evidence in the dC dataset for age-dependent viral load distribution. The log-evidence ratio for the dC dataset explicitly favored the dependent model, but it decreased when y’min was considered the lower threshold. The log-evidence ratio was low for the reverse causal relation y → x (viral load influences age), indicating no robust y → x structure in the data.
The log-evidence ratios for the dL dataset for either threshold favored an independent model. The authors performed data randomizations multiple times to generate several randomized datasets. Randomizations were repeated to validate and calibrate evidence ratio computation. The log-evidence ratios between causal (dependent) and independent models for 10 randomized datasets were much lower than for the original datasets.
Next, the authors investigated whether the age difference(s) in viral load distribution would be relevant for infection dynamics. To this end, viral load was linked to infectivity, the probability of transmitting infection. The team used a probit distribution-based ‘projected virus isolation success’ as a proxy for infectivity. There were no larger differences in the projected infectivity across different age groups. This meant that, at maximum, a 50% difference (more likely a smaller one) in infectivity could be anticipated due to differential viral loads across age groups.
Conclusions
In summary, the authors found that differences in SARS-CoV-2 viral load distribution across age groups in the dC dataset were statistically significant. They observed a statistically significant increase in viral load with age, a trend that fits the generally accepted notion of a weaker immune response as age advances.
As such, its impact on the infectivity of different age groups was moderate. Overall, the findings underscored that viral load was only modestly age-dependent, concordant with evidence from the literature. The authors suggested that the described models could be adapted easily for general purposes and may be used for future SARS-CoV-2 variants or pandemics.
- Guardiani, M. et al. (2022) "Causal, Bayesian, & non-parametric modeling of the SARS-CoV-2 viral load distribution vs. patient’s age", PLOS ONE, 17(10), p. e0275011. doi: 10.1371/journal.pone.0275011. https://journals.plos.org/plosone/article?id=10.1371/journal.pone.0275011
Posted in: Medical Science News | Medical Research News | Disease/Infection News
Tags: Adolescents, Children, Coronavirus, Coronavirus Disease COVID-19, covid-19, CT, Immune Response, Labor, Mortality, Polymerase, Polymerase Chain Reaction, Research, Respiratory, RNA, SARS, SARS-CoV-2, Severe Acute Respiratory, Severe Acute Respiratory Syndrome, Syndrome, Transcription, Virology, Virus
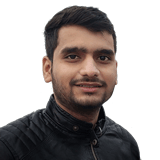
Written by
Tarun Sai Lomte
Tarun is a writer based in Hyderabad, India. He has a Master’s degree in Biotechnology from the University of Hyderabad and is enthusiastic about scientific research. He enjoys reading research papers and literature reviews and is passionate about writing.
Source: Read Full Article