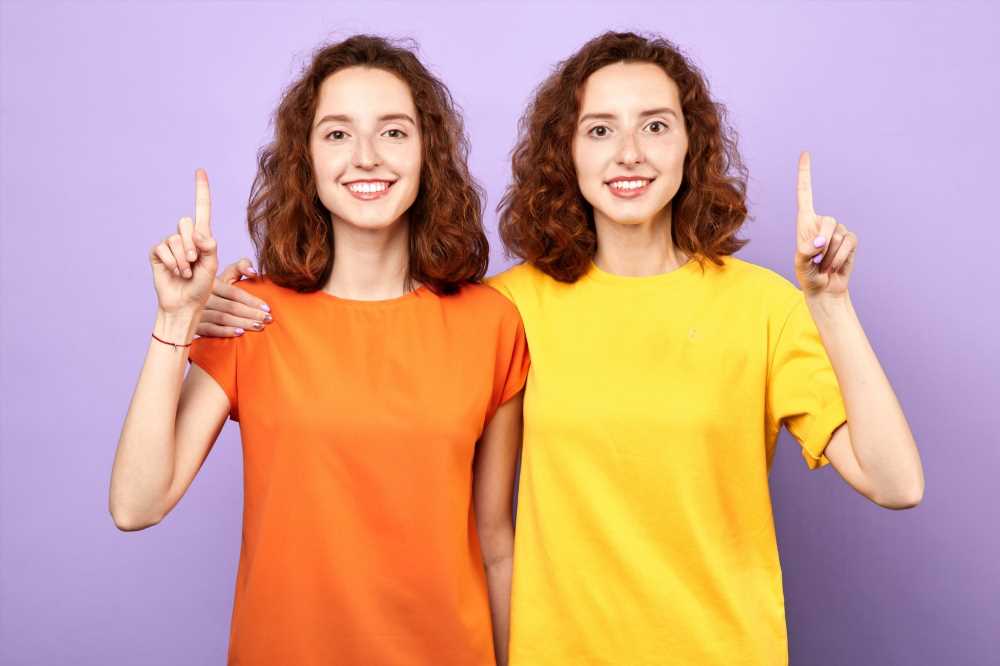
In a recent study published in Cell Reports, researchers identified look-alike humans, who were genetically unrelated, using facial recognition (FR) algorithms for multi-omics studies.
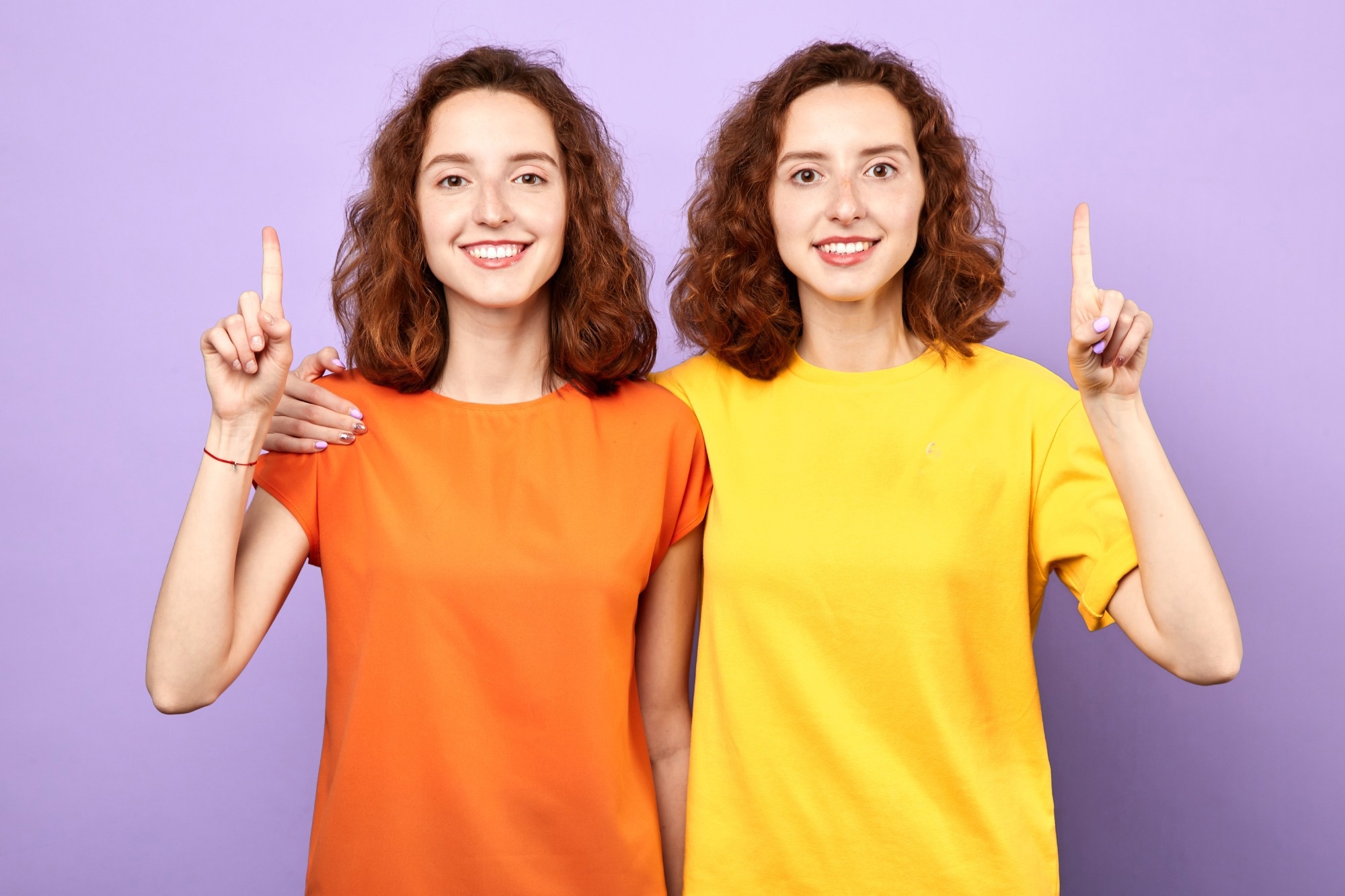
Study: Look-alike humans identified by facial recognition algorithms show genetic similarities. Image Credit: The Faces/Shutterstock
Background
Historically, research into face morphology was based on craniofacial anomalies. These days, smartphones and closed-circuit television (CCTV) cameras use facial recognition software, igniting interest in normal-range face variations.
Since it is difficult to find the right human sample, studies have not adequately characterized random human beings that objectively share facial features. Once found, this unique set of humans could facilitate studying how genomics, epigenomics, and microbiomics contribute to a resemblance between humans.
About the study
In the present study, researchers obtained headshot pictures of 32 look-alike people from the photographs taken by François Brunelle, a Canadian artist. First, they determined an objective measure of “likeness” for these look-alike pairs. Next, they used the custom deep convolutional neural network Custom-Net, the MatConvNet algorithm, and the Microsoft Oxford Project face API (three methods) to yield variable results.
As a control for a high similarity score, the team ran facial recognition algorithms on the photographs of monozygotic twins obtained from the University of Notre Dame twins database. The researchers analyzed the deoxyribonucleic acid (DNA) extracted from the saliva of all 32 look-alike humans by multi-omics to obtain three levels of biological information:
i) genomic,
ii) epigenomic, and
iii) microbiome.
For the genomic information, the researchers ran single nucleotide polymorphisms (SNP) microarray that interrogated 4,327,108 genetic variants selected from the International HapMap and 1,000 Genomes Projects and targeted genetic variation down to 1% minor allele frequency (MAF). Furthermore, they evaluated the face gene enrichment in 19,277 SNPs of 3,730 genes by applying a hypergeometric test and a 10,000 iterations Monte Carlo simulation.
For epigenetic analyses, the researchers used a DNA methylation microarray that evaluated over 0.85 million 5'—cytosine—phosphate—guanine—3' (CpG) sites. They calculated the absolute age differences for 16 look-alike pairs according to their chronological and epigenetic age, i.e., per their date of birth and DNA methylation clock, respectively.
For microbiome analysis, they performed ribosomal ribonucleic acid (RNA) direct sequencing. Lastly, the team examined 68 biometrics and lifestyle attributes for all look-alike human pairs.
Study findings
The authors noted that all three FR systems clustered 16 of the 32 (50%) look-alike doubles, and their similarity scores were similar to those obtained from monozygotic twins according to MatConvNet. Conversely, only one pair clustered (6.2%) from 16 look-alike cases that did not cluster by the three FR networks. Genomic analyses revealed nine of 16 (56.2%) look-alike pairs clustered together in the unsupervised clustering heatmap with bootstrap; thus, they considered them 'ultra' look-alikes.
The principal-component analysis (PCA) and t-distributed stochastic neighbor embedding (t-SNE) showed that these 'ultra' look-alikes also had a genotyping resemblance. Related to population stratification, among the 16 look-alike pairs, 13 were of European ancestry, one East Asian, one Hispanic, and one Central-South Asian. Seven of the 13 White look-alike doubles did not cluster genetically, indicating alternative purposes for shared genetic variation between look-alike pairs.
The number of shared SNP positions was significantly higher than random non-look-alike pairs. In no iteration of random gene sets did facial genes exceed the number of face genes represented in the 19,277 SNP selection. The 1,794 face genes in 19,277 SNP selection constituted 26% of all the face genes in the study array (hypergeometric test p: 6.31e−172; Monte Carlo empirical p < 1e−4). Upon adding the reported face-associated SNPs to 19,277 SNPs, the authors observed that 11 of the 16 (68.7%) look-alike pairs clustered together, adding two new doubles. Genomic analyses clustered only one couple together per copy number variation (CNVs). Three look-alike pairs shared three CNVs, including a locus in chromosome 11 that target genes involved in craniofacial dysmorphic features [e.g., hydrolethalus syndrome 1 (HYLS1)].
The aging process changes facial morphology, and DNA methylation, a proxy for biological age, can or cannot be directly related to chronological age. However, it is associated with genetic variations in humans. Despite evidence for epigenetic variation in human populations, only one look-alike pair is clustered by DNA methylation. This pair was also clustered by SNPs, suggesting that the resembling epigenetic profile was likely due to their underlining shared genetics, also supported by analyzing CpGs in the vicinity of the SNPs.
In addition, ultra-look-alike pairs showed similar epigenetics. Three of the nine ultra-look-alike couples clustered within a window of +100 base pairs from the 19,277 SNPs. Thus, DNA methylation, as a marker of biological age and methylation quantitative trait loci (meQTL), could also show phenotypic similarities in ultra-look-alikes.
Regarding alpha diversity, based on the type of bacteria in the oral samples, only one look-alike pair clustered together. However, this couple did not cluster together in SNP genotyping. From a quantitative standpoint, based on the amount of bacteria strands in the samples, again, only one look-alike pair clustered together (6.25%, 1/16). Microbiome analysis clustered one look-alike pair, but ultra-look-alike pairs had similar weights; thus, indicating microbiome composition could be related to obesity.
Conclusions
Taken together, the study findings support a significant role of genetic, epigenetic, and microbiome components in determining human facial features. Intriguingly, the same biological determinants are responsible for human physical and behavioral attributes. Thus, the study data could provide a molecular basis for future applications in areas of biomedicine, evolution, and forensics. However, future studies would require collaborative efforts to predict the human face structure based on the individuals' multi-omics landscape.
- Ricky S. Joshi, Maria Rigau, Carlos A. García-Prieto, et al. (2022). Look-alike humans identified by facial recognition algorithms show genetic similarities. Cell Reports. doi: https://doi.org/10.1016/j.celrep.2022.111257 https://www.cell.com/cell-reports/fulltext/S2211-1247(22)01075-0
Posted in: Medical Science News | Medical Research News | Miscellaneous News
Tags: Aging, Allele, Bacteria, Biomedicine, Cell, Chromosome, Chromosome 11, CpG, Craniofacial, Cytosine, DNA, DNA Methylation, Epigenetics, Epigenomics, Evolution, Forensics, Frequency, Gene, Genes, Genetic, Genetics, Genomic, Genomics, Genotyping, Guanine, Locus, Microarray, Microbiome, Morphology, Nucleotide, Obesity, Research, Ribonucleic Acid, RNA, Single Nucleotide Polymorphisms, Syndrome, Twins
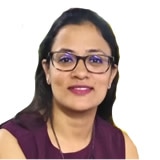
Written by
Neha Mathur
Neha is a digital marketing professional based in Gurugram, India. She has a Master’s degree from the University of Rajasthan with a specialization in Biotechnology in 2008. She has experience in pre-clinical research as part of her research project in The Department of Toxicology at the prestigious Central Drug Research Institute (CDRI), Lucknow, India. She also holds a certification in C++ programming.
Source: Read Full Article